A new neural network developed by Facebook’s VR/AR research division could enable console-quality graphics on future standalone headsets.
This ‘Neural Supersampling’ algorithm can take a low resolution rendered frame and upscale it 16x. That means, for example, a future headset could theoretically drive dual 3K panels by only rendering 1K per eye – without even requiring eye tracking.
Being able to render at lower resolution means more GPU power is free to run detailed shaders and advanced effects, which could bridge the gap from mobile to console VR. To be clear, this can’t turn a mobile chip into a PlayStation, but it should bridge the gap somewhat.
“AI upscaling” algorithms have become popular in the last few years, with some websites even letting users upload any image on their PC or phone to be upscaled. Given enough training data, they can produce a significantly more detailed output than traditional upscaling. While just a few years ago “Zoom and Enhance” was used to mock those falsely believing computers could do this, machine learning has made it reality. The algorithm is technically only “hallucinating” what it expects the missing detail should look like, but in many cases there is little practical difference.
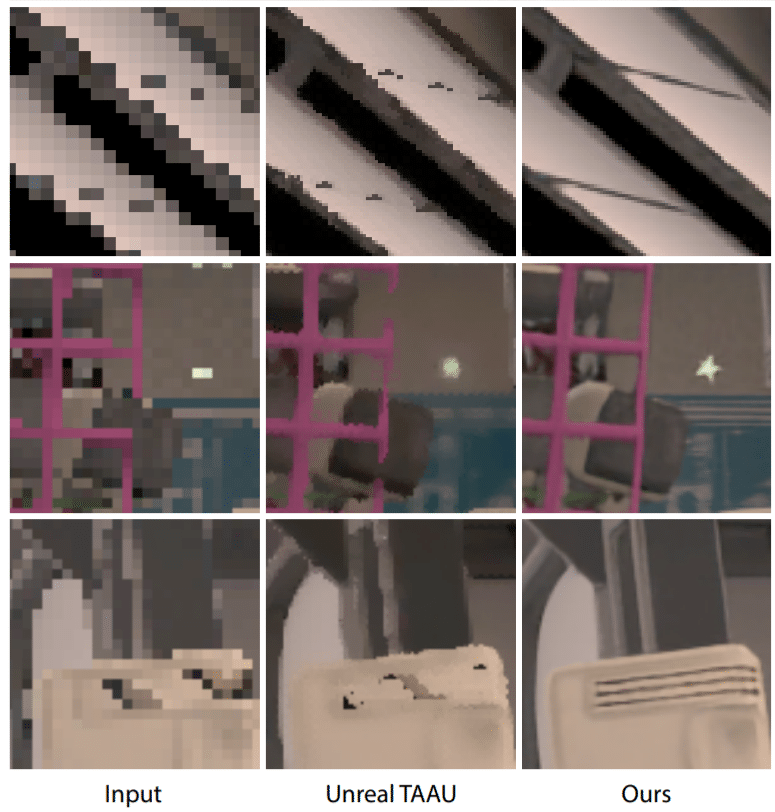
Facebook claims its neural network is state of the art, outperforming all other similar algorithms- the reason it’s able to achieve 16x upscaling. What makes this possible is the inherent knowledge of the depth of each object in the scene- it would not be anywhere near as effective with flat images.
In the provided example images, Facebook’s algorithm seems to have reached the point where it can reconstruct even fine details like line or mesh patterns.
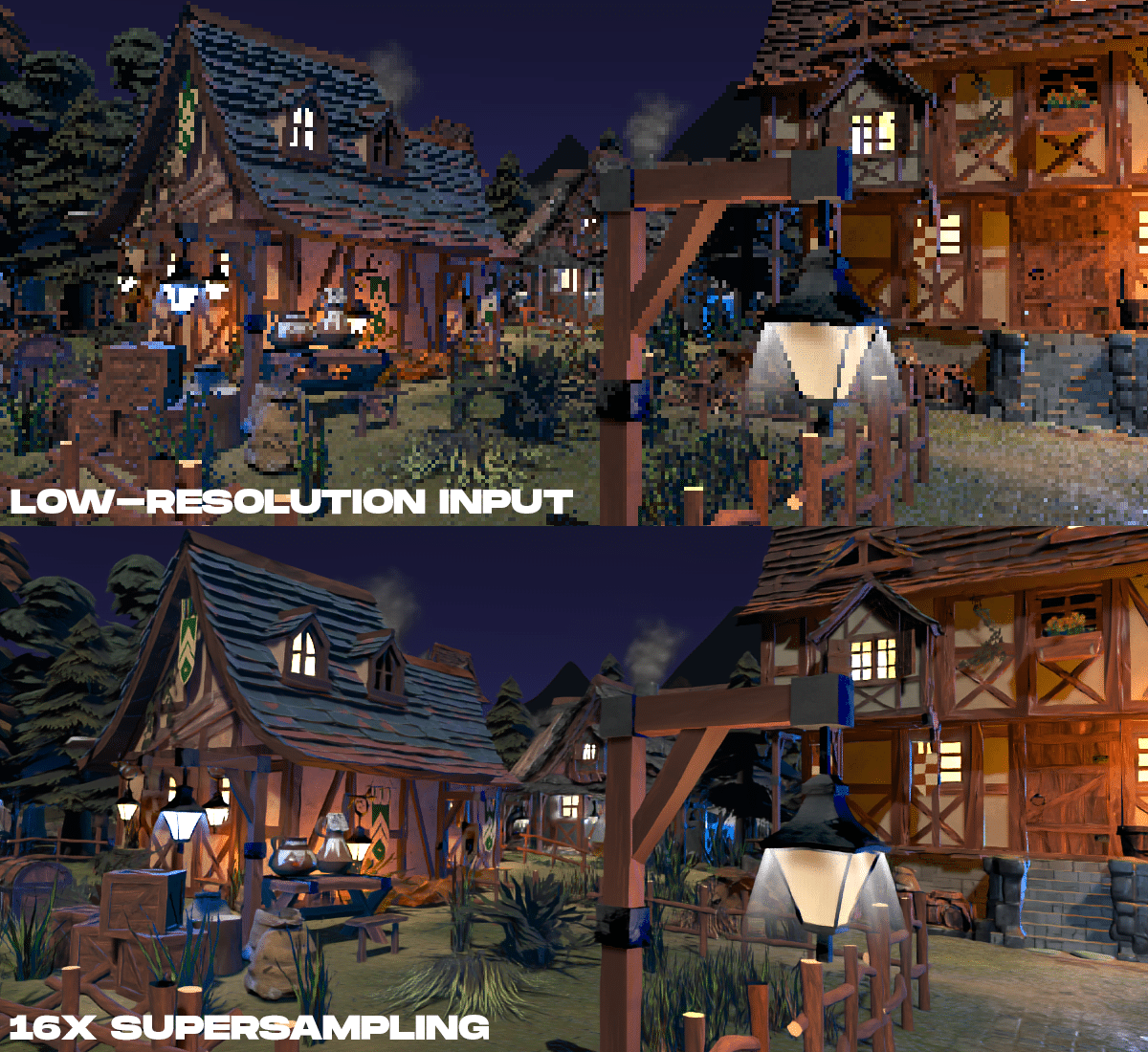
Back in March, Facebook published a somewhat similar paper. It also described the idea of freeing up GPU power by using neural upsampling. But that wasn’t actually what the paper was about. The researchers’ direct goal was to figure a “framework” for running machine learning algorithms in real time within the current rendering pipeline (with low latency), which they achieved. Combining that framework with this neural network could make this technology practical.
“As AR/VR displays reach toward higher resolutions, faster frame rates, and enhanced photorealism, neural supersampling methods may be key for reproducing sharp details by inferring them from scene data, rather than directly rendering them. This work points toward a future for high-resolution VR that isn’t just about the displays, but also the algorithms required to practically drive them,” a blog post by Lei Xiao explains.
For now, this is all just research and you can read the paper here. What’s stopping this from being a software update for your Oculus Quest tomorrow? The neural network itself takes time to process. The current version runs at 40 frames per second at Quest resolution on the $3000 NVIDIA Titan V.
But in machine learning, optimization comes second- and happens to an extreme degree. Just three years ago, the algorithm Google Assistant uses to speak realistically also required a $3000 GPU. Today it runs locally on several smartphones.
The researchers “believe the method can be significantly faster with further network optimization, hardware acceleration and professional grade engineering”. Hardware acceleration for machine learning tasks is available on the Snapdragon chips - Qualcomm claims its new XR2 has 11x the ML performance as Quest.
If optimization and models built for mobile system-on-a-chip neural processing units don’t work out, another approach is a custom chip designed for the task. This approach was taken on the $50 Nest Mini speaker (the cheapest device with local Google Assistant). Facebook is reportedly working with Samsung on custom chips for AR glasses, but there’s no indication of the same happening for VR- at least not yet.
Facebook classes this kind of approach “neural rendering”. Just like neural photography helped bridged the gap between smartphones and dedicated professional cameras, Facebook hopes it can one day push a little more power out of mobile chips than anyone might have expected.