VR took another big Jump forward (sorry, had to) with their announcement at Google IO yesterday. The Jump platform promises to completely reshape the VR content production landscape, from capture to display. Understanding how and why requires really grokking Jump itself. So based on screenshots and published specs, we’ll do our best.
First, Jump is a physical platform. It’s a GoPro array, like you might get from 360 Heroes or any one of a dozen other GoPro-holder companies. Google VP of Product Management and head of VR/AR Clay Bavor claimed the rig works with ‘off the shelf cameras,’ and the presentation implied it was camera agnostic, but practically speaking it’s a GoPro rig. Google isn’t selling it, but they are releasing the CAD drawings so anyone can manufacture them. Here’s a rendering, but they also had photos, so this isn’t vaporware:
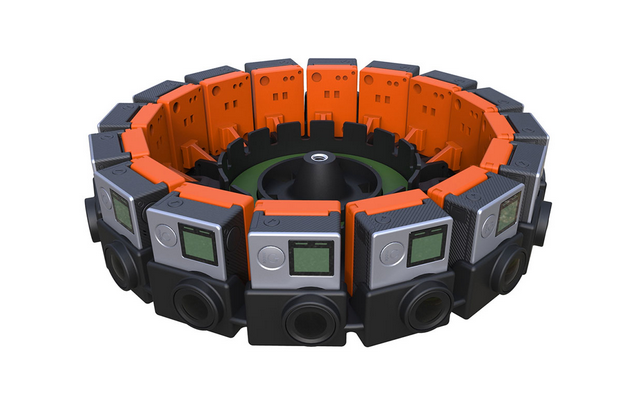
And yes, they even made one out of cardboard ($8,000 worth of camera and 8 cents worth of cardboard, like a Ferrari engine in, well, a car like mine). But before you go start a company manufacturing these things, know that GoPro is also going to sell this Jump array, supposedly by ‘this summer.’ GoPro has been pretty good at hitting published delivery dates, if not speculated ones, so this is probably accurate. But you can still make your own if you want it in a different color, say, or a different material. I can almost guarantee there will be a half-dozen carbon fiber versions available right away, for example, and plastic ones will be on Alibaba pretty much instantly. Just keep in mind: any rig probably has to be built to tight tolerances, because the camera positions are important. See below, where we discuss ‘calibrated camera arrays.’
At first glance, the rig is a little bigger than equivalent GoPro holders. It holds 16 cameras, and there are no up-facing or down-facing cameras at all. Based on the GoPro 4 Black’s specs, the rig captures a full 360 laterally, but just over 120 degrees vertically. So it will be missing about 30 degrees around each pole, directly above your head and at your feet. (Probably not a big deal, I think — but maybe a small differentiator for one of the existing companies? Like Mao’s Great Leap, Google’s is going to create a few casualties.) Also, Jump is arranged like a mono rig, where each camera is pointed in a different direction. Most 3D GoPro rigs have two cameras pointed in each direction like this:
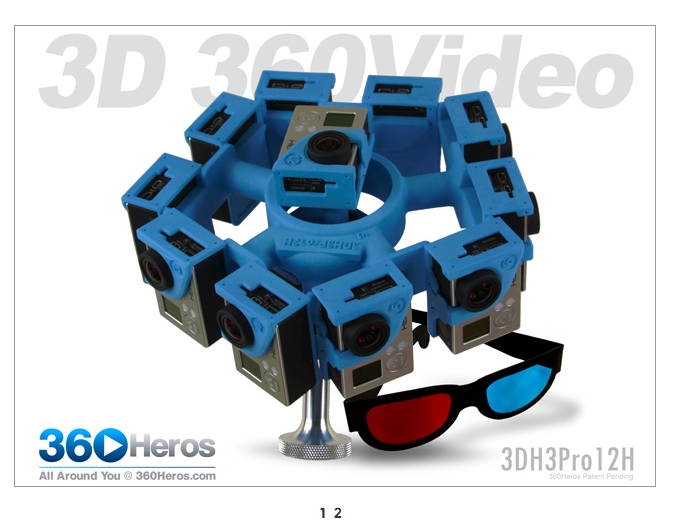
By having two cameras pointed in each direction, you get a different view for each eye, which gives you parallax and your 3D effect. You also get seams, because the ‘left’ camera pointing in one direction is pretty far from the ‘left’ camera facing another direction. There’s an entire micro industry built around painting together the seams left by that arrangement (and the smaller seams in mono 360, though that’s usually more tractable).
But this is where Google blows everything else out of the water: their rig is a 3D rig. But the cameras aren’t dedicated left eye or right eye; each camera captures pixels that are used for both eyes. Google gets their 3D effect the same way Jaunt does, by using machine vision to infer 3D positions, then remapping those 3D positions so there is no seam. None. (In theory.) That’s a big deal, because now with $10k in semi-professional gear you can produce flawless 360 3D VR. That’s amazing. (It’s also not the whole story — I’ll discuss limitations shortly.)
Here’s how it works: because their software knows exactly where each camera is relative to the other, if they can find a patch of pixels that matches between two cameras, they can figure out where that point must be in 3D space in the real world. Using that 3D data, they can bend and warp the footage to give you what’s known as a ‘stereo-orthogonal’ view: basically, each vertical strip of footage has exactly the correct parallax. For normal rigs, that’s only the case with subject matter directly between two of the taking lenses, and it falls off with the cosine of the angle that the subject matter makes with the lens axis. (In other words, the further you are off to the side, the less stereo depth you normally have.) Now, this requires a ton of computation, but Google can do this pretty fast — probably faster than existing off-the-shelf stitchers — because they are dictating how and where you put the cameras.
Here’s the deal, in depth (sorry again): when you shift from one camera in the array to another, the perspective shifts by a little bit. To stitch the images (or build a 3D model), you find matching regions in the image from adjacent cameras. These are called ‘point correspondences.’ So if you start with a few pixels from camera A, and you’re looking in camera B’s footage for a similar patch of pixels, you actually know where to start and which direction to start looking in. This speeds up the search process massively, compared to an uncalibrated rig. To be fair, existing stitching software like Videostitch or Kolor (conveniently part of GoPro now) probably make some pretty good algorithmic assumptions about your camera rig — but the pure mathematical advantage of a calibrated rig is huge.
So, once you find the matching patches, and you know how far you had to ‘travel’ in the source image to find them, you can compute the distance to the camera array for that region. If the object is at infinity, it will be some small distance away in the two images (it’s not in the same region of the images because the cameras are pointed in slightly different directions). As the subject moves closer to the camera, that distance will drop to zero and then start to get larger as the subject approaches the rig. The exact distance in the images is fully dictated by the distance of the subject, so you can compute 3D positions with scary accuracy.
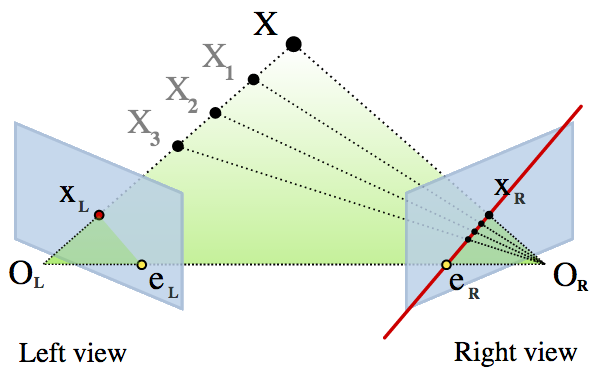
To be totally precise, you’re searching along epipolar lines in the second image. (Because GoPro lenses distort heavily, the lines are actually arcs, unless they’re remapped in memory… but that diagram would totally break my head.) Note that the hyper-efficient algorithms behind this are only a few years old; those interested in geeking out heavily should consider reading “Multiple View Geometry,” which covers both the specific case here and the general case of uncalibrated camera rigs. Available here; full disclosure, I’ve only read the first couple hundred pages, but I probably read each page ten times. It’s dense, and you’ll spend some time trying to visualize things like ‘planes at infinity.’ Plus it’s heavy on linear algebra; enjoy.
More about what this all means after the jump….
Continued on page 2…
So, with a 3D model, you can even get some amount of look-around, head-tracking style. Note that it’s only a little bit, and with Jump you have absolutely zero data about vertical parallax: so you can move your head left and right within the boundary of the rig and get new perspectives, but if you move up and down you’ll start to lose pixels to parallax. Image points will theoretically move correctly, though — you do have proper 3D information, you just don’t have the background pixels that would come into view as objects parallax past each other with your vertical movements. (Interpolating pixels for in-painting is not impossible, but you don’t necessarily get this out of the box with Jump. Because I have to nitpick every technology, that’s how this deal works.)
Now, I may have alluded to this before when discussing light fields, but machine vision isn’t perfect. Shoot, human vision isn’t perfect, but at least we’re used to its idiosyncrasies. But the problem with this machine vision technique is it requires those little patches of pixels to look roughly the same from camera to camera. For totally matte surfaces with no specular reflections, this is substantially true. (Called ‘Lambertian‘ in technical language.) But anything that’s got some shine or glimmer won’t look right. There’s a reason the demo footage was pretty static, frankly — a lot of the outside world doesn’t play so nicely with machine vision techniques. And I bet those motorcycles would’ve looked odd, or at least the reflections in them would’ve, if the cameras were closer.
Another thing that can screw the algorithms up is a highly repetitive pattern, because the algorithm may find false matches. (Brick walls and chain link fences are the bane of this technique, though there are ways around it.) Also, featureless regions are tough to match, and glass is brutal. There are also ‘non-things’ that show up in an image, like the intersection between telephone lines: while there will be regions that look similar in two views, they don’t correspond to any physical thing. In the case of the telephone lines, where they appear to cross is a function of your perspective, there’s no point in 3D space that is the ‘crossing point’. But with this technique the computer will think there’s a match, think that it’s seeing a physical cross in the world, not two tricks of perspective. Compression artifacts create some pretty brutal high frequency errors, too — remember, GoPros compress on the fly to h.264, and the quality of that compression is highly variable. And don’t even get me started on foliage, or worst of all: lens flares. There were flares in the footage, but you wouldn’t have noticed them because they’re caused by the large, soft fluorescent lights, so the flares have soft edges and blend nicely between views. Hard lights like the sun won’t make such well-behaved flares, though.
Then again, based on all the machines Google has staring at Street View footage, I think it’s safe to say there’s probably no one better at solving these problems. In fact, the demo gave some clues about how they’re doing this. In a flourish of showmanship, Bavor played a slide of algorithmic ‘tracing’ along the contours of the 3D scene:
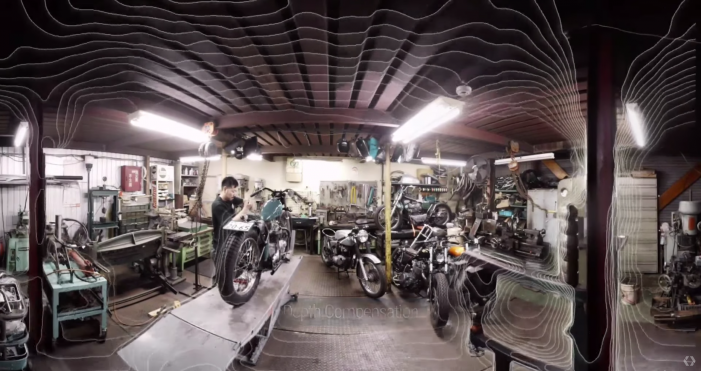
And this hints at what Google may be doing to solve a lot of the challenges I cited earlier: when you have a patch you can’t match properly, look nearby and just interpolate the depth data from the surroundings. And if you get something totally spurious, like a lens flare or false match on telephone wires, ignore it and interpolate around it. The result would be a ‘softening,’ sort of a low-passing of the contour map. And I believe the traces we saw were an accurate representation of the algorithm’s output, too, because it really didn’t match the scene geometry all that closely; the lines appeared too smooth around edges (look at the top of the pillars), and were in all places continuous, like a huge rubber sheet pushed over the top of the scene. (If that sounds crazy inaccurate, remember that we get a lot of our depth perception by combining many depth cues; avoiding grossly incorrect stereo disparity is more important than getting it precisely right… which is why I’ve always thought the focus on ‘stereo-orthogonality’ was a little overstated for narrow fields of view, but I digress.) If this is in fact the output of the algorithm, one region in particular shows this rubber-sheet effect really strongly:
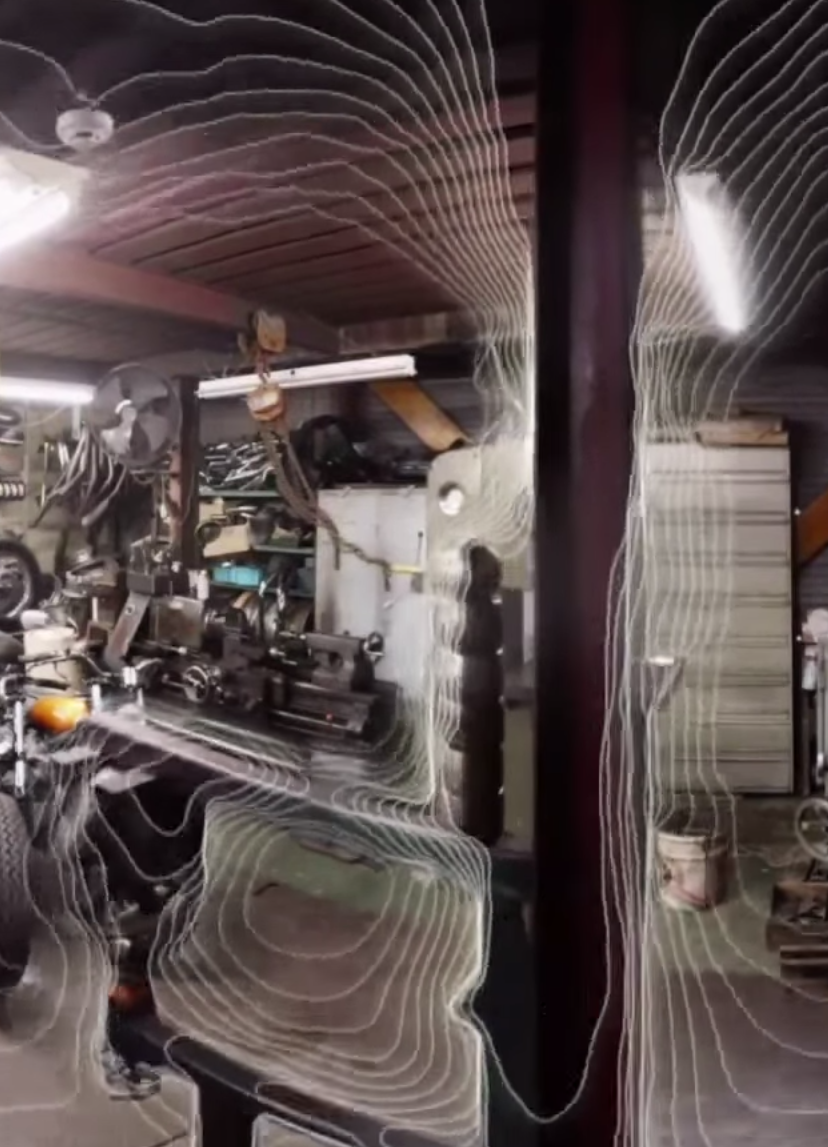
Nonetheless, there will be artifacts. In fact, the demo footage did have some rather visible artifacts if you were looking (and I’m always looking). If you focus on the dies stacked on a press in the center right foreground, their reflections appear to double and shear as you ‘translate’ from side to side during the lateral motion demo. There’s also a curiously doubling motorcycle wheel on the floor behind the press, but that’s a little tougher to see in a screen grab.
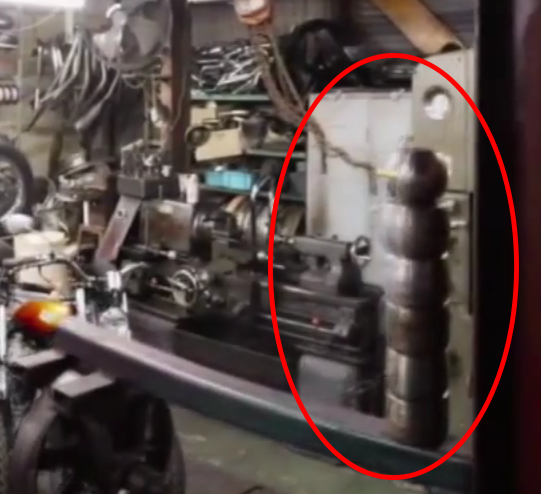
Okay, so that’s super tiny and forgivable — ‘it’s not even an area I care about,’ I can hear you saying (plus it was during a lookaround demo). But please stop being so forgiving, , because until proven otherwise, this system will suffer from issues with the usual suspects: reflections, water, repeating patterns, translucency, transparency, etc. One motorcycle shop demo, impressive though it may be, isn’t the ultimate acid test of the technology. That said: man, it looked pretty darn good, so I’m confident the Jump will be a major component of VR production starting very soon. At a bare minimum, this raises the bar significantly for GoPro VR production, which some SVVR panelists estimated is about 80% of the live action VR production going on today.
So what will it be like to use this rig? It’s pretty bulky. GoPro is evidently supporting it though, so hopefully triggering all the cameras will be pretty easy, and Bavor said the exposure would match (and presumably shutter and color temperature, though the demo involved a ‘calibration’ step to make the footage match better; hopefully that won’t be necessary with the shipping version). Supposedly we’re getting ‘frame sync’ too. Because Bavor wasn’t speaking technically, I don’t know whether ‘frame sync’ means within-half-a-frame sync, or something more like millisecond or microsecond sync, which is what you get with professional 3D camera systems. I have heard a rumor that the way the GoPro 3 and 4 are designed, they can’t be hard synced to the milli- or microsecond scale, though this is hearsay and could be completely wrong. (For what it’s worth, GoPro had this system for 3D in the past, but there’s no option for the Hero 3 or Hero 4… so there may be something to this rumor.) From a content producer’s standpoint, this could actually be a problem; I’ve seen major stitching and correspondence errors with high-motion subjects or fast-moving cameras when there is as little as half of a millisecond of jitter, and the camera clocks are probably just crystal oscillators which are very prone to drift (I’ve even seen parts-per-thousand drift, which would mean dropping a frame every 20 seconds or so at 60 fps). Granted, the accuracy you needs depends entirely on how fast your subject matter is moving, of course, but it’s a little ironic that GoPros might not be the best choice for action footage. (Yes, I really did just write that.)
In the same vein, the GoPro has a noticeable rolling shutter. It appears that the maximum frame rate dictates the rolling shutter, and you can safely assume it’s nearly 100% rolling if you’re shooting at or near the max frame rate for a given resolution setting. In slightly less technical terms, the harder you push the camera and the lower your frame rate the more jello you’ll get in your shot. When 30 fps is max — for example, for 4k footage on the GoPro Black — that means you’re looking at about a 30 millisecond rolling shutter. Any camera movement causes problems, and fast-moving subjects will distort. How will that distortion play with Google’s machine vision techniques? Unclear. I’m sure we’ll learn more this summer.
Of course, for that reason and others, the system may work better at one of the lower-resolution, higher frame rate GoPro settings. Say, 1920×1440 at 60 fps, which will give you a faster rolling shutter (still probably on the order of 12 msec, which is not good but not terrible). And of course, VR footage looks a lot better at higher frame rates, so there’s another reason to bump up to 60p.
And if it sounds like I’m being nitpicky, I am — that’s sort of my MO with camera technology. To be frank, I was blown away by the demo; you should watch the video yourself, though. Me, I even signed up for the summer beta test program, here. Give it a whirl, just as long as you’re behind me in line. (Personal aside to Clay Bavor: feel free to put me on the short list. I’m just sayin’.)
But how is it to use, again? Frankly, that’s a lot of cameras and data to wrangle. In our last article about Jump, we calculated that array would produce approximately 430 GB/hour. Here’s how I figure it: with ProTune enabled on a GoPro Black, you can record to an h.264 compressed stream at 60 Mbit. That’s about 7.5 megabytes per camera per second. Over an hour, 16 cameras * 3600 seconds / hour * 7.5 megabytes / camera-second adds up to… 432 Gigabytes. (Sharp inhale of breath.) Storage is cheap and all, but it looks like the cost of this rig isn’t going to be the cameras, it’s hard drives. And processors. Oh, and microSD cards. Keeping track of 16 pinkie-nail-sized cards is no small task. On proper digital film sets, there’s literally a role called ‘data wrangler,’ for the poor saps that have to keep footage backed up and the cameras rolling. They’re probably going to hate Jump.
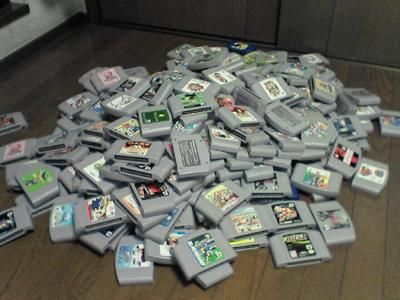
Bavor mentioned casually that the raw image those cameras create is ‘as large as five 4k TVs.’ As long as we’re doing finger-counting math, I’d say that’s about 20k, or 20480 x 2160. (Oculus was right, by the way: your computer is not fast enough.) This strongly implies you’re shooting at 1440, by the way: divide that resolution by 16 cameras and it comes out to exactly 1920 x 1440. (This is probably right, but the coincidence is more likely than it seems, since all resolutions are small number multiples of HD resolutions, they tend to divide pretty evenly… particularly with 16 cameras.) There’s currently no word on how large the output file is, but at this point I’d suspect it’s roughly equivalent to existing VR, or maybe a little higher: call it 4k or so, with most of the capture resolution being redundant pixels for the computers to chomp on.
How redundant? Well, if we lined the GoPros up so their images didn’t overlap, they would cover 1510 degrees (94.4 degrees VFOV x 16 cameras). Since we only need to cover 360 degrees, that’s a coverage ratio of more than 4:1. In other words, most image points are actually being seen by more than two cameras, which is ample fodder for the machine vision techniques, and may allow for things like flare rejection if two cameras agree and the third (or fourth) has an imaging artifact. Again, that’s assuming we’re right about the resolution settings, but the numbers do add up.
Of course, if we’re shooting at, say, 1920 x 1440, we actually don’t quite have 2160 vertical pixels — remember the cameras are sideways, but even so we’re short of these projections by ~240 pixels. Of course, the cameras don’t cover 180 vertical degrees anyway, so the vertical pixels/degree is almost what it would be for 2160p video stretched to 180… aaaaand we’re officially splitting hairs of a yet-unpublished spec, based on a throwaway line in a keynote. (That’s what we do here; you’re welcome.)
So, unless you do high end VR already, or shoot digital IMAX, or work in large-format printing, you probably aren’t too familiar with resolutions that high. And, despite sounding snarky, I can honestly only assume that resolution is completely wasted on most Google Cardboard users, unless those headset upgrades are substantial. So either this is future-proofing (possible; 4k upload was enabled on YouTube long before anyone was watching it), or Google is planning to make YouTube the premiere destination for video on every headset on the market.
In other words, Google just announced themselves as major players in the VR content space. And while the landscape of live action VR is still pretty uncertain, Google has staked out a sizable claim.